您现在的位置是:How to make sense of AI neural network: A new study reveals >>正文
How to make sense of AI neural network: A new study reveals
上海品茶网 - 夜上海最新论坛社区 - 上海千花论坛3人已围观
简介By subscribing, you agree to our Terms of Use and Policies You may unsubscribe at any time.Artificia...
By subscribing, you agree to our Terms of Use and Policies You may unsubscribe at any time.
Artificial neural networks are like digital versions of our brains. They learn from data, not rules, and they can perform extraordinary tasks, from translating languages to playing chess. But how do they do it? What is the logic behind their calculations? And how can we trust them to be safe and reliable?
AI brains: How do they work?
These are some of the questions that puzzle both computer scientists and neuroscientists. The latter also face a similar challenge in understanding how the human brain works. How do the billions of neurons in our head produce our thoughts, emotions, and decisions? Despite years of research and medical advances, we still need to learn more about the brain.
See Also Related- China accelerates launch of large-language models as Elon Musk visits Beijing
- ChatGPT: Unveiling the power of advanced language models
- AI language models show political biases, new research shows
- Meta developing an AI model more powerful than LLaMa 2
Fortunately, artificial neural networks are more accessible to study than biological ones. We can measure the activity of every neuron in the network, manipulate them by turning them on or off, and see how the network responds to different inputs.
However, this approach has a limitation: the individual neurons do not have a clear meaning or function. For instance, a single neuron in a small language model can be active in various situations, such as when it sees academic references, English conversations, web requests, or Korean texts. In a vision model, a single neuron can react to cat faces and car fronts. The same neuron can have different meanings depending on the context.
A new paper by researchers from former OpenAI brains working at Anthropic proposes a better way to understand artificial neural networks. Instead of looking at individual neurons, they look at combinations of neurons that form patterns or features. These features are more specific and consistent than neurons, and they can capture different aspects of the network's behavior.
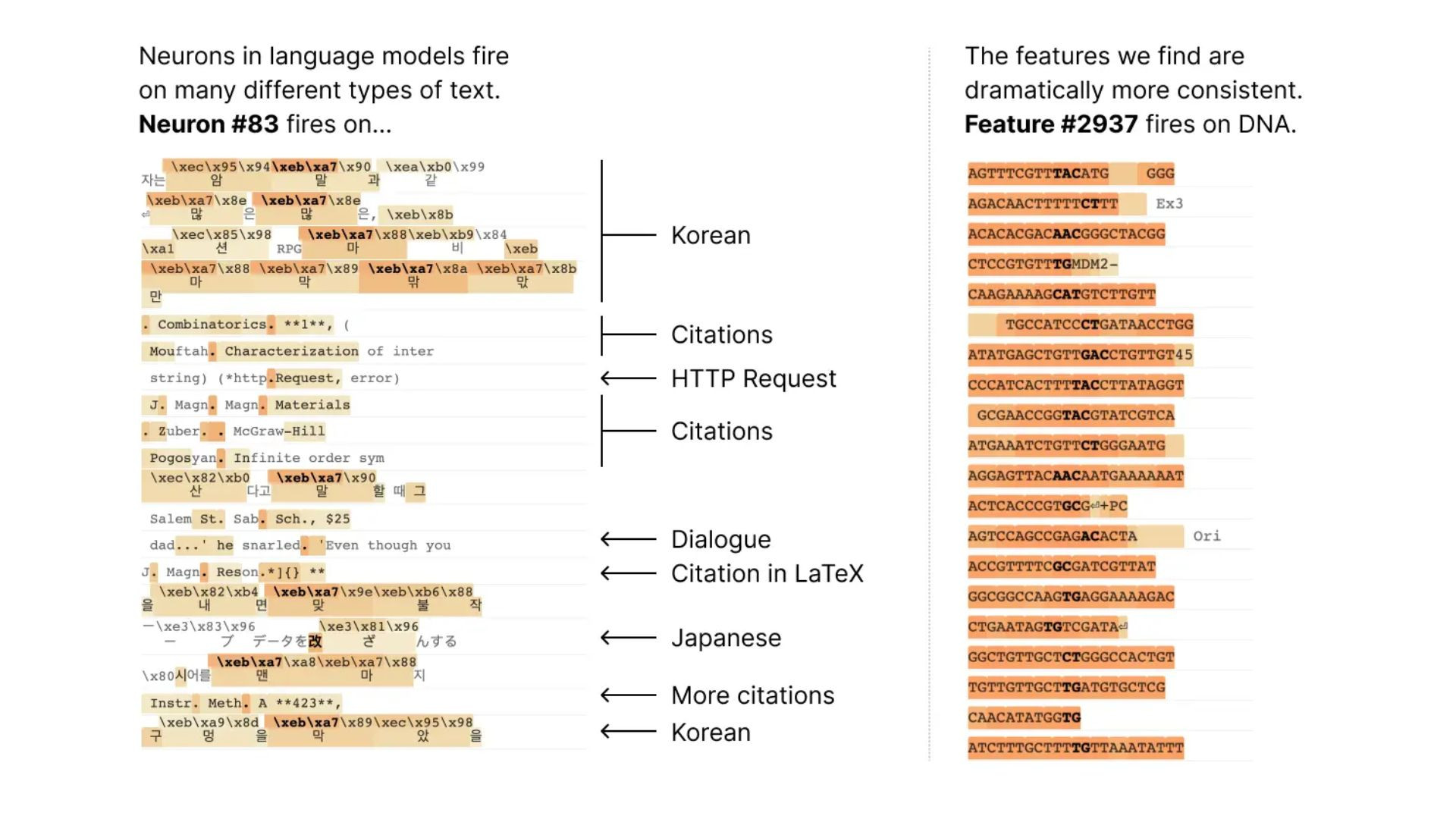
Anthropic
The paper, Towards Monosemanticity: Decomposing Language Models With Dictionary Learning, shows how to find these features in small transformer models widely used for natural language processing. The researchers use dictionary learning to decompose a layer with 512 neurons into more than 4,000 features. These features represent diverse topics and concepts, such as DNA sequences, legal terms, web requests, Hebrew texts, nutrition facts, etc. Most of these features are hidden when we only look at the neurons.
Features
The researchers use two methods to prove that the features are more interpretable than the neurons. First, they ask a human evaluator to rate how easy it is to understand what each feature does. The features (red) score higher than the neurons (teal). Second, they use a large language model to generate short descriptions of each feature and then use another model to predict how much each feature is activated based on the report. Again, the features perform better than the neurons.
The features also allow them to control the network's behavior more precisely. As shown below, by activating a feature artificially, they can make the network produce different outputs that match the feature's meaning.
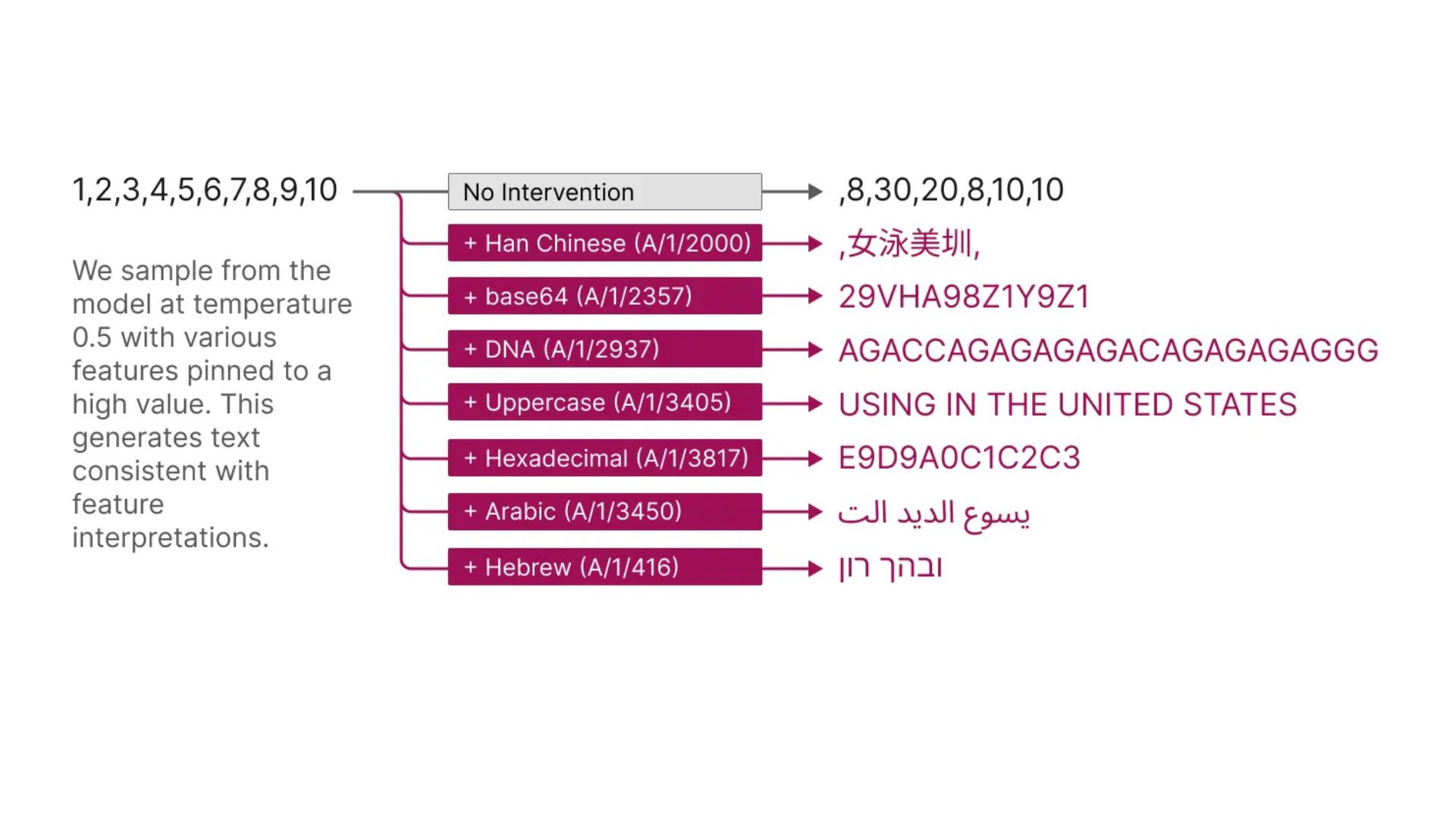
Anthropic
But this is not the end of the story. The researchers also zoom out and examine the feature set as a whole. They find that the features learned are universal between different models, so the lessons learned by studying the features in one model may generalize to others. They also experiment with tuning the number of features they know. They find this provides a "knob" for varying the resolution at which we see the model: decomposing the model into a small set of features offers a coarse view that is easier to understand, and decomposing it into a large group of features provides a more refined view revealing subtle model properties.
This work results from Anthropic's investment in Mechanistic Interpretability – one of their longest-term research bets on AI safety. Until now, the fact that individual neurons were uninterpretable presented a severe roadblock to a mechanistic understanding of language models. Decomposing groups of neurons into interpretable features has the potential to move past that roadblock. They hope this will eventually enable them to monitor and steer model behavior from the inside, improving the safety and reliability essential for enterprise and societal adoption.
Their next challenge is to scale this approach from the small model they successfully use to frontier models, which are many times larger and substantially more complicated. For the first time, they feel that engineering is the next primary obstacle to interpreting large language models rather than science.
This study opens up new possibilities for understanding and improving artificial neural networks. It also bridges the gap between computer science and neuroscience, as both fields share similar goals and challenges in deciphering complex systems. By learning from each other's methods and insights, we can unlock more natural and artificial intelligence secrets.
To learn more about you can read their paper, Towards Monosemanticity: Decomposing Language Models With Dictionary Learning.
Tags:
转载:欢迎各位朋友分享到网络,但转载请说明文章出处“上海品茶网 - 夜上海最新论坛社区 - 上海千花论坛”。http://www.jz08.com.cn/news/57847.html
相关文章
Waves (WAVES) hits record high – What do indicators say
How to make sense of AI neural network: A new study revealsWaves (WAVES)has hit record highs in a recent bullish run that appears to be stronger than ever. The...
阅读更多
10 Most Promising Cryptocurrencies to Buy & Hold in 2022
How to make sense of AI neural network: A new study revealsCryptocurrencies have not had a good start of the year. According to data compiled by CoinGecko show...
阅读更多
Memeinator’s stage three presale nears completion: Should you buy now?
How to make sense of AI neural network: A new study revealsKey takeawaysMemeinator’s stage three presale will soon be over as the team has raised over $6...
阅读更多
热门文章
- More Than 75 Crypto Exchanges Have Closed This Year
- BlockFi's Bitcoin Trust to compete with GBTC
- Global Marketing Head At Coinbase Exits The Company
- Cryptocurrency price update: Dogecoin, Polkadot
- Bitcoin has been forked more than 400 times since 2009
- Bitcoin found support at $25k (again). YTD performance remains impressive.